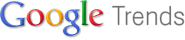
There is a very interesting research on using publicly available Google Trends data to predict realized stock market volatility. The researches use a number of standard models of realized volatility and add search query index results to come up with better forecasts. The most interesting part that I found was that the authors claim significant gain (over standard models) in prediction accuracy during high volatility period in the autumn of 2008. Unfortunately the analysis does not extend to the most recent period of high volatility.
Abstract:
This paper studies the dynamics of stock market volatility and retail investor attention measured by internet search queries. We find a strong co-movement of stock market indices' realized volatility and the search queries for their names. Furthermore, Granger causality is bi-directional: high searches follow high volatility, and high volatility follows high searches. Using the latter feedback effect to predict volatility we find that search queries contain additional information about market volatility. They help to improve volatility forecasts in-sample and out-of-sample as well as for different forecasting horizons. Search queries are particularly useful to predict volatility in high-volatility phases.I have been working on a similar approach, also using Google Trends and Google Correlate to find drivers for volatility. While intuition was telling me that keywords like "bearish", "negative", "crash", "risk", "drawdown", or just "volatility" would have high predictive power - they did not, it was mostly benign searches like "stock futures" and various references to stock indexes that were most correlated to volatility. I guess people search more when market is crashing, not so much when there is a bull market. I am very interested in advancing research on this topic, and hope to share some of my own findings soon.
A similar topic is analysed in Web search queries can predict stock market volumes, link by a team of researches using proprietary company-specific search query dataset from Yahoo!. They observe one-way causality, that search queries help predict trading volume, but not the reverse.
Abstract:
We live in a computerized and networked society where many of our actions leave a digital trace and affect other people’s actions. This has lead to the emergence of a new data-driven research field: mathematical methods of computer science, statistical physics and sociometry provide insights on a wide range of disciplines ranging from social science to human mobility. A recent important discovery is that query volumes (i.e., the number of requests submitted by users to search engines on the www) can be used to track and, in some cases, to anticipate the dynamics of social phenomena. Successful exemples include unemployment levels, car and home sales, and epidemics spreading. Few recent works applied this approach to stock prices and market sentiment. However, it remains unclear if trends in financial markets can be anticipated by the collective wisdom of on-line users on the web. Here we show that trading volumes of stocks traded in NASDAQ-100 are correlated with the volumes of queries related to the same stocks. In particular, query volumes anticipate in many cases peaks of trading by one day or more. Our analysis is carried out on a unique dataset of queries, submitted to an important web search engine, which enable us to investigate also the user behavior. We show that the query volume dynamics emerges from the collective but seemingly uncoordinated activity of many users. These findings contribute to the debate on the identification of early warnings of financial systemic risk, based on the activity of users of the www.