Few weeks ago Dr Jonathan Kinlay from Quantitative Research and Trading blog published a series of excellent articles on volatility. I wanted to review and comment on the notes.
Forecasting Volatility in the S&P500 Index
Modeling Asset Volatility
Long Memory and Regime Shifts in Asset Volatility
Range-Based EGARCH Option Pricing Models
There are four main articles that discuss practical volatility forecasting topics. The material is not new; it was published around fifteen years ago by Dr Kinlay's previous funds, Caissa Capital, Investment Analytics, and later Proteom Capital.
As I understand Kinlay was the idea generator behind volatility trading, and Proteom Capital had some excellent years in 2003 and 2004, and that was pretty much it. Managers typically don't close funds because of great performance, but I don't know what actually happened; if you have any information, please send me an email.
Regardless, the published research is important, and I believe worthwhile to pay attention to. I will also comment on some more recent updates.
Forecasting Volatility in the S&P500 Index
tl;dr : Arfima-Garch, straddles trading.
my comment: While we know that autocorrelation of volatility decays very slowly, Arfima-Garch is actually pretty bad at predicting volatility. Midas and HAR(X) type models using realized volatility (and jumps, or other factors) have been demonstrated to be perform much better. The weakness of Garch seems to come from both the form and MLE estimation 'issues' but that's a separate topic.
Modeling Asset Volatility
tl;dr : volatility exhibits complex dynamics - long memory, momentum, and mean reversion
my comment: The most interesting part is the last paragraph - "Dispersion" This is not dispersion in the index vs names sense, at least not exactly. The idea (if I understand correctly) that both first and second moment relationships can be used to construct cointegrated baskets of options. Not sure how it is supposed to work in practice - do you hedge gammas or not?
Long Memory and Regime Shifts in Asset Volatility
tl;dr : remember all that stuff about long memory? actually, it could be structural breaks, and no long memory
my comment: structural breaks can confound models, luckily there are break test available. This is an import point, don't skip this one.
Range-Based EGARCH Option Pricing Models
tl;dr :2 factor Egarch, but on ranges instead of squared returns.
my comment: 20 years ago having ability to store and process tick data was uncommon, and calculating realized volatility was not possible. Therefore models were developed based on 'newspaper data' - daily open, high, low, close. Range Egarch has several features that make it better than let's say garch -
1, range being far better vol estimator than squared returns,
2 - log vol is much better behaved, and makes estimation more robust,
3 - two components in the model, fast and slow vol.
Range Egarch was probably one of the best way to create decent vol models back then, but of course better methods exists today. Now all production models feature these three elements ( robust estimate of vol, power transformation / generalized error, multiple scales ) in one way or another.
Subscribe to:
Post Comments (Atom)
Weekly market report
Wall st delivered a mixed bag of news with VIX, VNKY, and VSTOXX and their underlying markets almost unchanged. VXD - volatility index based...
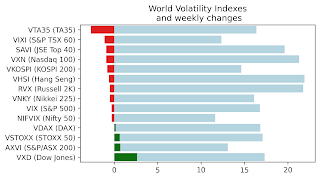
-
As I am sure all of you know Russia has began a full scale war against my home country Ukraine. Please make no mistake - Putin's goal ...
-
Wall st delivered a mixed bag of news with VIX, VNKY, and VSTOXX and their underlying markets almost unchanged. VXD - volatility index based...
-
Many investors are looking at VIX and VSTOXX indexes as a leading indicators of volatility in equity markets, however many are confused by t...
No comments:
Post a Comment